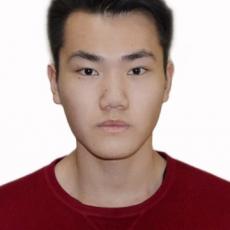
Saffron, a costly food ingredient and colorant, is susceptible to adulteration, which is when less expensive or effective materials are substituted in order to reduce costs or increase profits. The objective of this work is to improve classification and quantification accuracy by data fusion. Data fusion is combining multiple blocks of variables from different sensors together and has been applied in verification of food quality. In the present work, optical data and Raman spectroscopic data were fused in partial least squares discriminant analysis (PLS-DA) and partial least squares regression (PLSR) models to classify and quantify adulteration, respectively.
I applied a data fusion method to integrate optical color data and Raman spectroscopic data to improve the accuracy of classification and quantification of saffron adulteration. For this project, I conducted data collection, analysis, and summary remotely. It was challenging, for example, when I did the quantification using the specific software as there was no instruction or direct accessible resource to refer to, so I needed to find lots of background information and I tried to figure it out based on its principle by myself. Finally, with some luck, I was able to figure it out. Overall, this work demonstrated that data fusion can improve the accuracy of classification and quantification of saffron adulteration which will facilitate the rapid analysis of saffron quality and adulteration on site.
The spectroscopy and data fusion I used are only small parts of the whole food science field. Currently, I can only imagine the close future, like going to graduate school and doing some research on food chemistry. Although, I cannot determine what kind of work I will do after graduation, I always hold great interests in real world applications of food science. Food safety will be a main target of my future career.